Unleashing the Power of Data Annotation Tools for Business Growth
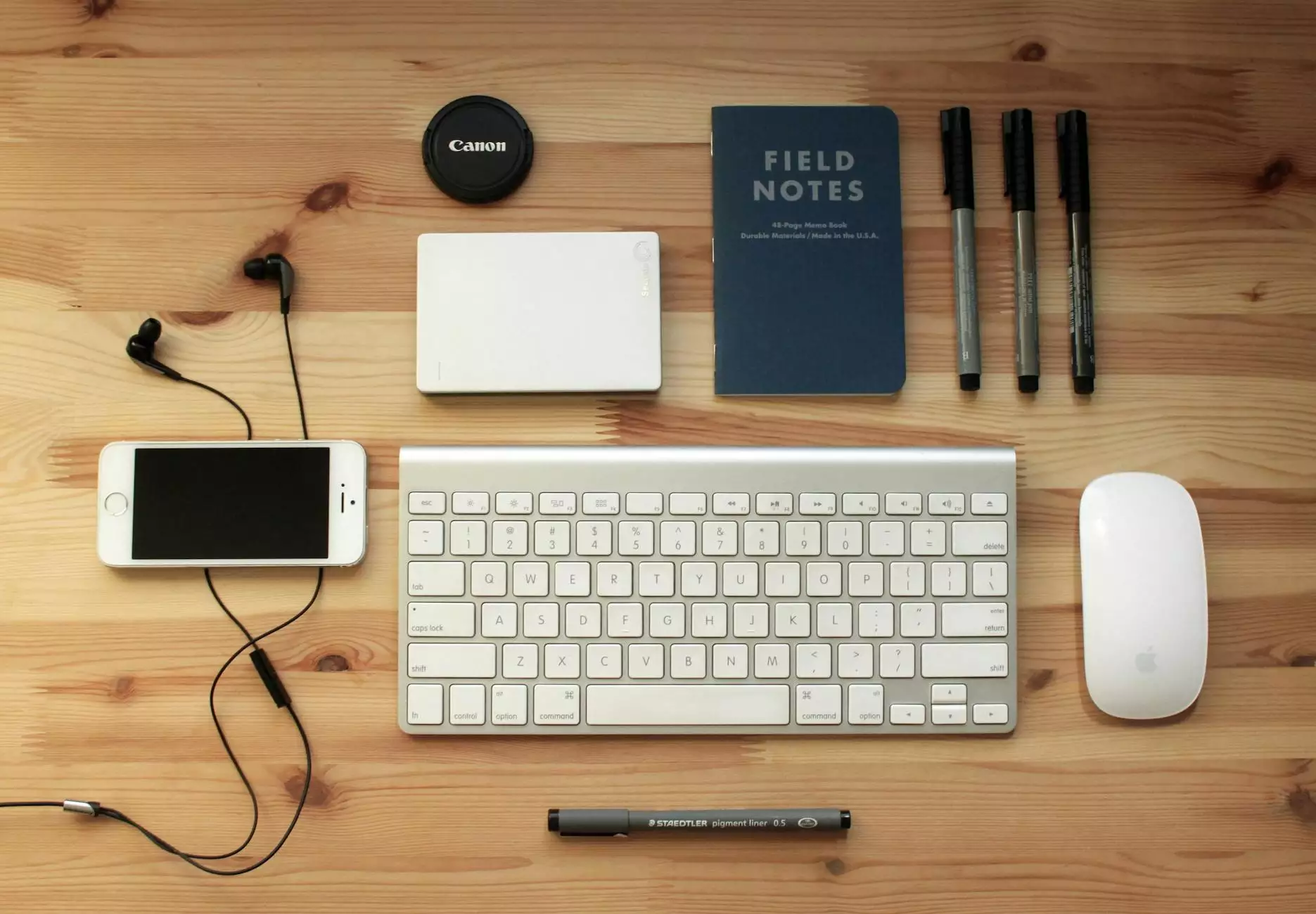
In the age of big data and artificial intelligence, data annotation tools have become indispensable resources for businesses aiming to harness the full potential of their data. As AI models become more sophisticated, the requirement for accurately labeled data has never been greater. In this comprehensive guide, we will explore the essentials of data annotation, its significance, and the tools that can elevate your business performance.
Understanding Data Annotation
Data annotation refers to the process of labeling or tagging data - whether that's images, text, audio, or video - so that machine learning models can learn and make predictions based on this information. The quality and accuracy of the labeled data directly impact the effectiveness of AI and machine learning applications.
Types of Data Annotation
Various types of data annotation are employed depending on the data type and the intended application:
- Image Annotation: This involves identifying and tagging objects within an image. It is crucial for applications like autonomous vehicles, where understanding the surroundings is essential.
- Text Annotation: This includes labeling sections of text for sentiment analysis, named entity recognition, and other NLP tasks.
- Audio Annotation: Here, audio clips are transcribed, labeled, or tagged for speech recognition tasks.
- Video Annotation: This process entails identifying actions, objects, or events in videos which is useful for security and surveillance systems.
The Importance of Data Annotation Tools
With the exponential growth of data, companies are increasingly relying on data annotation tools to automate and streamline the labeling process. Here’s why these tools are essential:
- Improved Efficiency: Manual data labeling is time-consuming and prone to errors. Data annotation tools expedite the process, allowing businesses to label vast datasets quickly and accurately.
- Cost-Effectiveness: By reducing the time and labor costs associated with manual annotation, businesses can allocate resources more effectively.
- High-Quality Data: These tools often come with features that enhance data quality, such as collaborative annotation, quality assurance checks, and advanced algorithms that suggest labels.
- Scalability: As data volume grows, businesses can easily scale their annotation efforts with robust tools without a significant increase in manpower.
Choosing the Right Data Annotation Tool
When selecting a data annotation platform, businesses should consider several factors to ensure they choose a tool that aligns with their needs:
1. Type of Data Support
Ensure that the tool supports the specific type of data you intend to annotate, whether it's images, text, audio, or video.
2. User Interface and Experience
A user-friendly interface can significantly enhance productivity. Look for tools with intuitive designs that allow team members to navigate and complete tasks with ease.
3. Collaboration Features
In modern business environments, collaboration is key. Select tools that facilitate teamwork, allowing multiple users to annotate and review data simultaneously.
4. Integration Capabilities
Ensure that the annotation tool integrates well with other systems and platforms your business uses, such as machine learning frameworks and data management tools.
5. Customization Options
The ability to customize workflows or create specific annotation guidelines can enhance the relevance of the annotation to your unique business needs.
6. Support and Resources
Effective support can make a significant difference in your experience with the tool. Check for the availability of resources such as tutorials, customer support, and community forums.
Top Data Annotation Tools for Businesses
Here are some of the leading data annotation tools currently available in the market, catering to various needs:
- Labelbox: A comprehensive annotation platform with a focus on image and video data. It offers collaborative features and integrates seamlessly with machine learning workflows.
- Snorkel: Optimal for data programming, Snorkel allows users to create and manage training datasets in a semi-automated way.
- SuperAnnotate: This tool provides advanced capabilities for image and video annotation with robust management features for large datasets.
- Scale AI: Known for its high-quality annotations and rapid turnaround, Scale AI serves many enterprise-level clients across industries.
- Amazon SageMaker Ground Truth: An integrated solution for building, training, and deploying machine learning models, it allows businesses to utilize machine-learning-assisted annotations.
Best Practices for Utilizing Data Annotation Tools
Leveraging data annotation tools effectively requires a strategic approach. Here are some best practices to follow:
1. Establish Clear Annotation Guidelines
Provide detailed instructions and examples to annotators to ensure consistency and accuracy in labeling.
2. Implement Quality Control Mechanisms
Utilize built-in quality assurance tools or employ a review team to verify the accuracy of annotations periodically.
3. Train Your Team
Ensure that your team is well-trained on both the data and the tools to maximize efficiency and reduce errors.
4. Monitor Progress and Metrics
Keep track of the annotation process through metrics such as time taken per task and accuracy rates to identify areas for improvement.
5. Stay Updated with Technology
Regularly update your annotation tools and methodologies to keep pace with advancements in AI and machine learning technologies.
The Future of Data Annotation in Business
As the landscape of data continues to evolve, so does the role of data annotation. The future promises greater integration of AI in the annotation process, with tools becoming smarter and more autonomous. This evolution is set to reduce manual labor further while enhancing data quality.
Moreover, companies that invest in robust data annotation tools will enjoy a significant competitive advantage, enabling them to process and analyze data more effectively. As the demand for machine learning applications escalates, so does the need for precise and well-annotated data.
Conclusion
In today's data-driven world, leveraging the right data annotation tool is crucial for businesses that wish to thrive and innovate. By understanding the importance of accurate data labeling, selecting the appropriate tools, and following best practices, organizations can harness the power of AI and machine learning, comprehensively transforming their operations.
To stay ahead in the competitive landscape, consider exploring solutions such as those provided by Keylabs.ai, which specializes in advanced data annotation and machine learning platforms.